1) Accuracy
The accuracy is the percentage of correct predictions:
\[accuracy = \frac{1}{n} \sum_{i=1}^{n} \unicode{x1D7D9} \{\hat y_i = y_i \}\]where $\hat y$ is the prediction and $y$ the true value.
Note: for imbalanced data, the accuracy can be very misleading because a classifier can achieve a high score just by predicting the majority class with probability 1.
In case of binary classification, the accuracy can be written as such: $accuracy = \frac{TP + TN}{TP + TN + FP + FN}$
2) True positive rate
The TPR measures the proportions of positives that are correctly identified.
\[TPR = \frac{TP}{P} = \frac{TP}{TP + FN}\]Note: this metric is typically used for medical tasks as it is crucial to minimize the count of false-negatives.
3) False positive rate
The FPR measures the proportions of negatives that are correctly identified.
\[FPR = \frac{FP}{N} = \frac{FP}{FP + TN}\]4) ROC (Receiver Operating Characteristic) curve
The ROC curve combines the $TPR$ and $FPR$.
Use of the ROC
If one model is used:
We use the ROC curve to evaluate the performance of one classifying model that we can obtain when varying a threshold.
If several models are used:
We use the ROC curve to compare several classifiers in evaluating the area under the curve (AUC) for a range of thresholds.
Intuition
After running the prediction of a specific model, we draw the confusion matrix (see below) with a certain threshold.
We then modify the threshold and draw another confusion matrix.
The ROC curve summarizes all of the confusion matrices that each threshold produced.
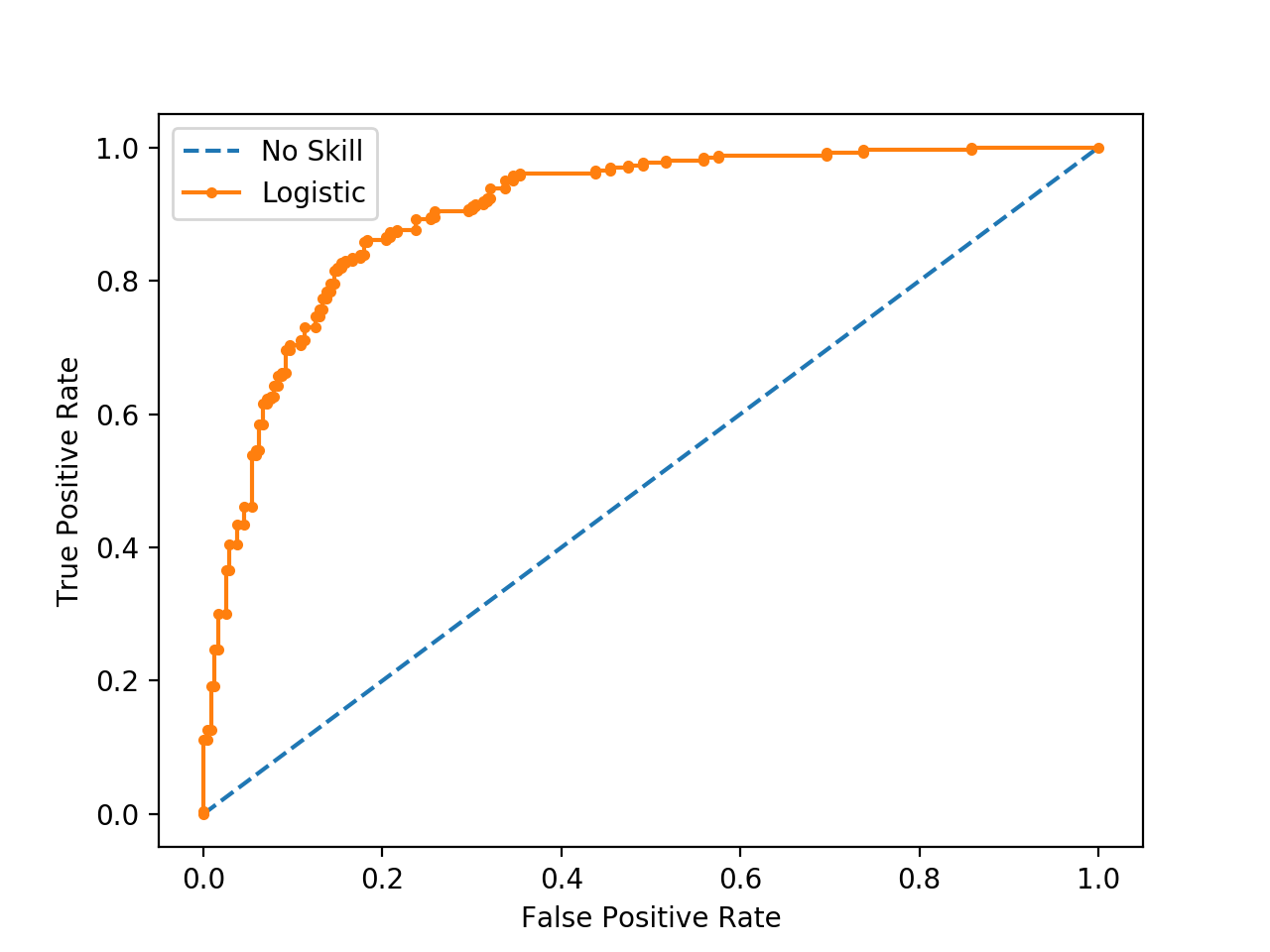
Implementation
-
Get probability predictions
-
Sort the probabilities (prediction)
-
Sort the validation (actual) according to previous sort
-
Loop on the sorted validation. At each iteration:
-
increment TP or FP
-
compute the TPR and FPR.
- Plot (FPR, TPR)
See this link for an implementation example. Below is also a computation of TPR from ChatGPT to better understand the relationship between thresholds and TPR:
prob = clf.predict_proba(X_test)
prob_pos = prob[:,1] # probabilities for the positive class
fpr, tpr, thresholds = metrics.roc_curve(y_test, prob_pos, pos_label=1)
recomputed_tpr = []
# Iterate through each threshold
for threshold in thresholds:
# Use the current threshold to determine the predicted labels based on probabilities
predicted_labels = np.where(prob_pos >= threshold, 1, 0)
# Calculate True Positives (TP), False Negatives (FN), False Positives (FP), and True Negatives (TN)
TP = np.sum((predicted_labels == 1) & (y_test == 1))
FN = np.sum((predicted_labels == 0) & (y_test == 1))
# Calculate True Positive Rate (TPR) for the current threshold
current_tpr = TP / (TP + FN) if (TP + FN) > 0 else 0.0
# Append the TPR value to the list
recomputed_tpr.append(current_tpr)
5) Precision
\[precision = \frac{TP}{TP + FP}\]Note: this metric should be used when false-negative is not too much a concern (e.g. YouTube recommendations, trading strategies). It can also be used when the data are imbalanced (see details below).
6) PR (Precision Recall) curve
The PR curve combines $TPR$ (recall) and $precision$.
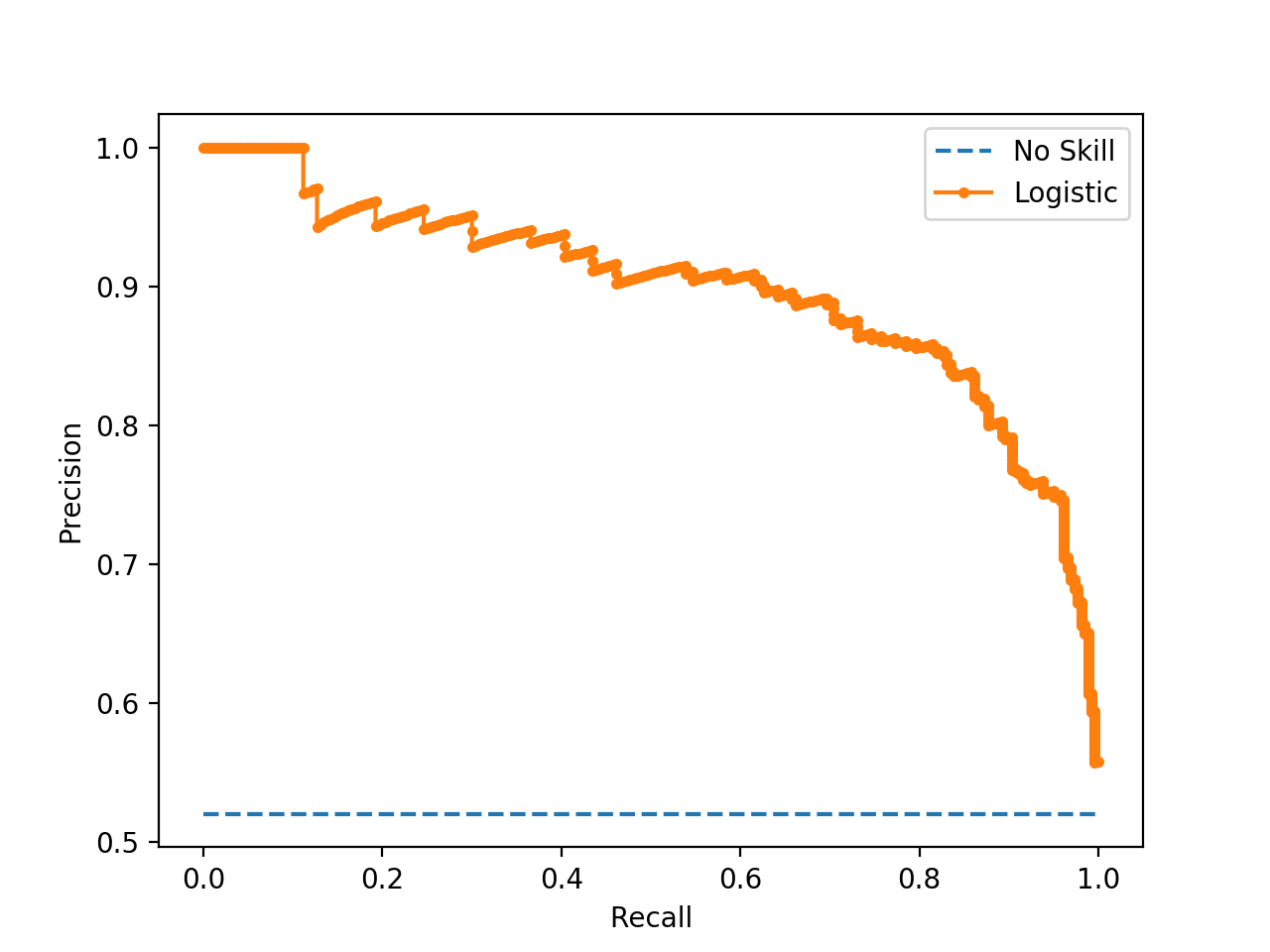
The PR curve is better adapted than the ROC curve in the case of imbalanced data:
ROC curve uses $FPR = \frac{FP}{\color{red}{N}} $ –> $N$ can be either very large or very small if classes are imbalanced.
PR curve uses $\text{Precision} = \frac{TP}{\color{green}{TP + FP}}$ –> the precision considers only the positive values coming from the model.
7) F1-score
F1-score is a single metric that combines TPR (recall) and precision.
\[f1 \text{-} score = 2\frac{precision*TPR}{precision+TPR}\]Note: it is widely used for imbalanced datasets since it involves the precision.
Metric summary
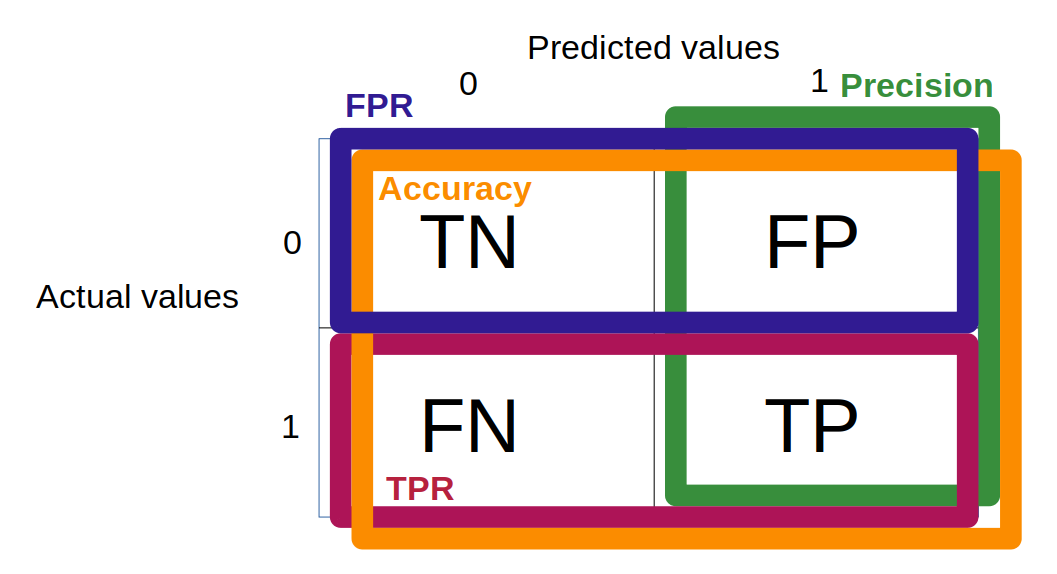