Neural network
Those notes are largely inspired by the tutorials of Andrew Ng.
While counting the layers, we usually don’t include the input layer. The first schema (left) is a 1-layer neural network whereas the second schema (right) is a 2-layers neural network. We notice that a 1-layer neural network doesn’t have any hidden layer.
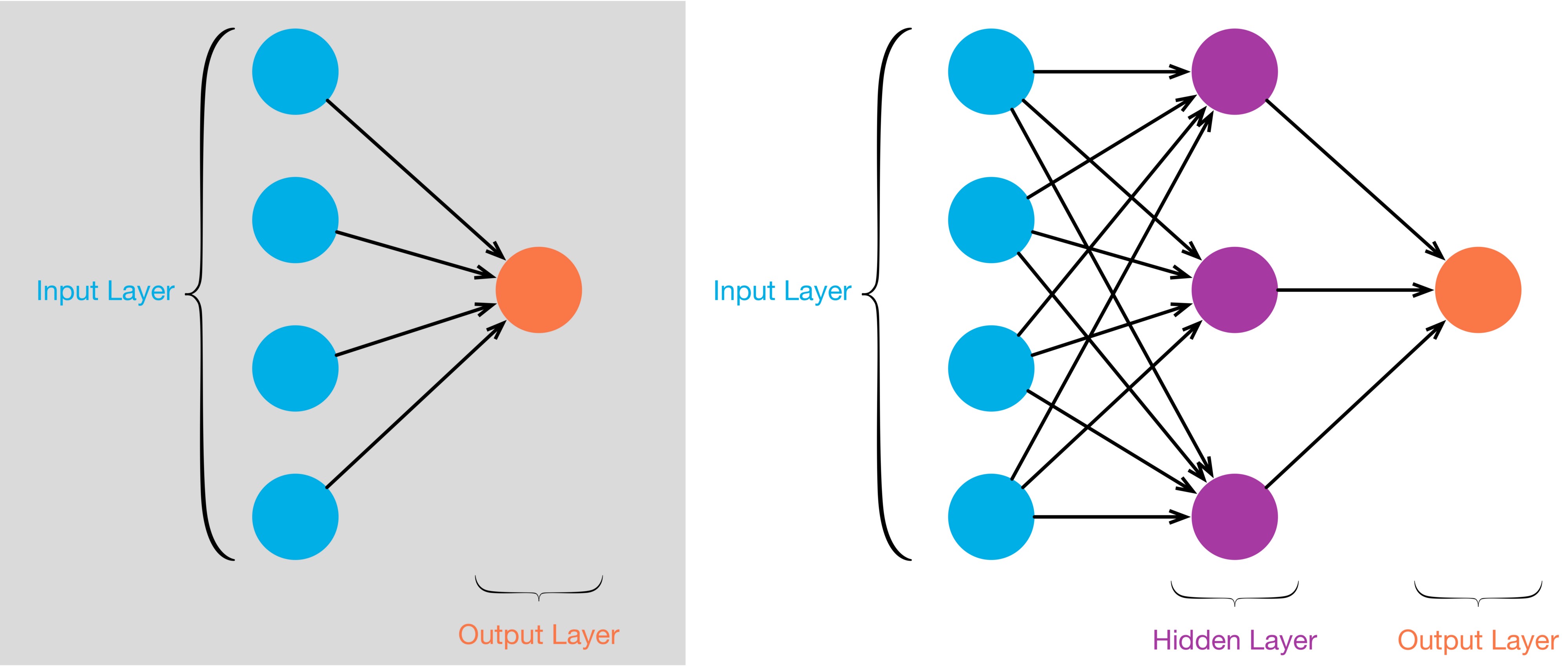
1-layer neural network
A 1-layer neural network can be seen as a logistic regression. The below explanation is largely inspired by the example from coursera (deeplearning.ai)
Let us take the example of an image that we want to classify in a binary way: man/woman
The picture is vectorized as a vector of pixels : \(\begin{pmatrix}x_1 \\ \vdots \\ x_p \end{pmatrix}\)
We use a regression to predict if it’s a man/woman:
$y=\omega^Tx + b$
Note: $x$ are all the pixels of one image.
We want a probability in output (if it’s $\ge 0.5$ then we say it’s a man).
We thus want the output to be $\widehat{y}=\sigma(\omega^Tx + b)=\mathbb{P}(y|x) \in [0,1]$
(see regression part to get more details on the sigmoid)
Now since it’s a binary classification, we want the $y$ (real value) to be $0$ or $1$.
Thus, the loss function is:
\[\mathcal{L}(y, \widehat{y})=-[y\log(\widehat{y})+(1-y)\log(1-\widehat{y})]\]We notice that the loss function decreases when $y \neq \widehat y$.
The cost function is the empiric loss on all examples:
\[J(\omega, b)=\frac{1}{m}\Sigma_{i=1}^m\mathcal{L}(\widehat{y}^{(i)}, y^i)\]Forward propagation
\[x, \omega, b \to z=\omega^Tx + b \to \widehat{y}=a=\sigma(z) \to \mathcal{L}(a,y)\]-
First arrow: regression
-
Second arrow: probability
-
Third arrow: error
Backward propagation
The idea is: with the error computed on the last step, we go backward in order to correct the parameters $\omega$ and $b$.
\[x, \omega ,b \leftarrow z=\omega + b \leftarrow \widehat{y}=a=\sigma(z) \leftarrow \mathcal{L}(a,y)\]To find the new value of $\omega$ we look for its change ($d \omega$) that we can obtain thanks to the Chain rule. It consists of decomposing the derivative into successive ones.
The parameters that are udpated during the training are the weights $\omega$ and the bias $b$. Thus we need to compute $\frac{d\mathcal{L}}{d\omega}=”d\omega”$ and $\frac{d\mathcal{L}}{db}=”db”$ .
First the weights:
\[d\omega=\frac{d\mathcal{L}}{da}\frac{da}{dz}\frac{dz}{d\omega}\] \[\text{where:}\] \[\frac{d\mathcal{L}}{da} = (\frac{d\mathcal{L}(a,y)}{da}) = -y \frac{1}{a} - (1-y) \frac{-1}{1-a} = \frac{1-y}{1-a} - \frac{y}{a} = \frac{(a-ay-y+ay)}{a(1-a)} = \frac{a-y}{a(1-a)}\] \[\frac{da}{dz} = \frac{d \sigma(z)}{dz} = \frac{-(-1)e^{-z}}{(1+e^{-z})^2} = \frac{e^{-z}}{1+e^{-z}} \frac{1}{1+e^{-z}}= (1-\sigma(z))\sigma(z) = (1-a)a~~~~\text{Reminder: } \sigma(z) = \frac{1}{1+e^{-z}}\] \[\frac{dz}{d\omega} = x\] \[\text{Thus:}\] \[\frac{d\mathcal{L}}{d\omega} = \frac{a-y}{a(1-a)}(1-a)ax = (a-y)x\]Then the bias:
\[db=\frac{d\mathcal{L}}{da}\frac{da}{dz}\frac{dz}{db}\] \[\frac{d\mathcal{L}}{da} = \text{same as above}\] \[\frac{da}{dz} = \text{same as above}\] \[\frac{dz}{db} = 1\] \[\text{Thus:}\] \[\frac{d\mathcal{L}}{db} = (a-y)\]Note: we can see that the updated weight depends on the previous prevision $a$. This is why it is necessary to recompute the predicted value at each iteration.
for i in range(num_iterations):
# Cost and gradient calculation
grads, cost = propagate(w, b, X_train, Y_train) # propagation on ALL the training sample
# Retrieve derivatives from grads
dw = grads["dw"]
db = grads["db"]
# update parameters
w = w - learning_rate * dw
b = b - learning_rate * db
# Record the costs
costs.append(cost)
def propagate(w, b, X, Y):
m = X.shape[1]
# FORWARD PROPAGATION (FROM X TO COST)
A = sigmoid(np.dot(w.T,X)+b)
cost = (- 1 / m) * np.sum(Y * np.log(A) + (1 - Y) * (np.log(1 - A))
# BACKWARD PROPAGATION (TO FIND GRAD)
dw = (1/m)*np.dot(X,(A-Y).T)
db = (1/m)*np.sum(A-Y)
2-layer neural network
Forward propagation

The main difference with the case of 1 layer is that now there are two sets of weights and biases. Moreover, the first set of weights is not a 1-column vector but a matrix.
Backward propagation

We now need to update 4 parameters: $W^{[1]}, b^{[2]}, W^{[2]}, b^{[2]}$.
For $W^{[2]}$:
\[dW^{[2]} =\frac{d\mathcal{L}}{da^{[2]}}\frac{da^{[2]}}{dz^{[2]}}\frac{dz^{[2]}}{dW^{[2]}} = \text{(similar logic as 1-layer)} = (a^{[2]}-y)a^{[1]T}\]For $b^{[2]}$:
\[db^{[2]}=\frac{d\mathcal{L}}{da^{[2]}}\frac{da^{[2]}}{dz^{[2]}}\frac{dz^{[2]}}{db^{[2]}} = \text{(similar logic as 1-layer)} = (a^{[2]}-y)\]For $W^{[1]}$:
\[dW^{[1]}=\frac{d\mathcal{L}}{da^{[1]}}\frac{da^{[1]}}{dz^{[1]}}\frac{dz^{[1]}}{dW^{[1]}}\] \[\text{where:}\] \[\frac{d\mathcal{L}}{da^{[1]}} = \color{red}{\frac{d\mathcal{L}}{dz^{[2]}}\frac{dz^{[2]}}{da^{[1]}}} = (a^{[2]}-y)W^{[2]}\] \[\frac{da^{[1]}}{dz^{[1]}} = \frac{d\sigma(z^{[1]})}{dz^{[1]}} = \text{(similar logic as 1-layer)} = (1-a^{[1]})a^{[1]}\] \[\frac{dz^{[1]}}{dW^{[1]}} = \text{(similar logic as 1-layer)} = x\] \[\text{finally:}\] \[dW^{[1]} = (a^{[2]}-y)W^{[2]}\color{red}{*}((1-a^{[1]})a^{[1]})x\]Warning: the element-wise product is necessary to have the right dimensions at the end.
For $b^{[1]}$:
\[db=\frac{d\mathcal{L}}{da^{[1]}}\frac{da^{[1]}}{dz^{[1]}}\frac{dz^{[1]}}{db^{[1]}} = (\text{similar logic as for }dW^{[1]}) = (a^{[2]}-y)W^{[2]}\color{red}{*}((1-a^{[1]})a^{[1]})\]